Day 19 cont ️
“The #LiberalParty has accidentally left part of its email provider’s #subscriber details exposed, revealing the types of #data harvested by the party during the #election campaign.
This gives rare #insight into some of the specific kinds of data the party is keeping on voters, including whether they are “predicted Chinese”, “predicted Jewish”, a “strong Liberal” and other #PersonalInformation.”
#AusPol / #DataScience / #inference / #voters / #Liberal / #LNP / #Nationals <https://www.crikey.com.au/2025/04/17/victorian-liberals-data-exposed-email-mailchimp-federal-election-crikey/>
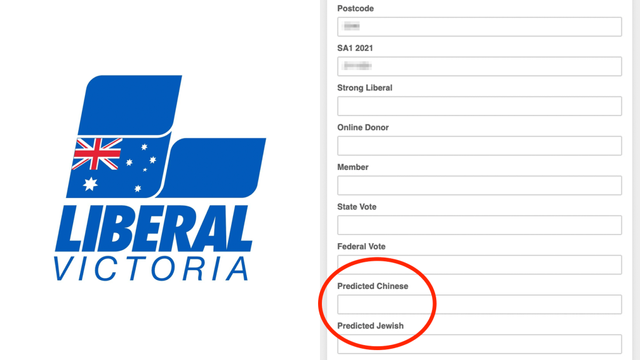